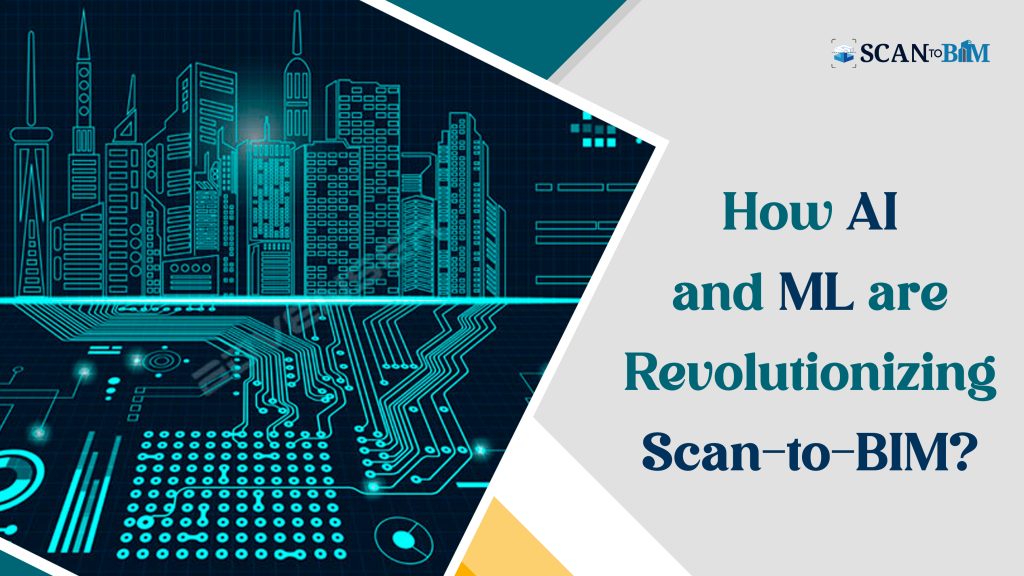
Introduction
Technological advancements have brought about a profound transformation in the construction and architecture sectors in recent years. One such transformation is the adoption of Scan to BIM Services. This process integrates laser scanning technology with BIM software to create accurate and comprehensive 3D models of existing buildings and infrastructure. However, the real game-changer in this process is the integration of Artificial Intelligence (AI) and Machine Learning (ML) algorithms. This blog post will explore how AI and ML empower the Scan-to-BIM process, making it faster, more accurate, and more efficient.
Understanding Scan-to-BIM
Before diving into the role of AI and ML, let’s briefly understand the Scan-to-BIM process itself. Scan-to-BIM involves the following key steps:
- Data Collection: This phase uses laser scanning technology to capture precise measurements and point cloud data of a physical structure or site. These scans generate vast amounts of data, which must be processed and converted into usable information.
- Data Processing: The collected point cloud data is processed to remove noise and outliers, resulting in a cleaner and more accurate dataset.
- Model Creation: This processed data is converted using BIM software into a 3D model that accurately represents the scanned structure. This model includes information about the building’s geometry, spatial relationships, and elements such as walls, floors, windows, and doors.
- Attribute Assignment: Additional information, such as material properties, component details, and maintenance data, is assigned to the model’s elements.
- Analysis and Documentation: The BIM model is analyzed for various purposes, including clash detection, structural analysis, and energy efficiency simulations. It is also used for creating detailed documentation and construction plans.
The Role of AI in Scan-to-BIM
Artificial Intelligence plays a critical role in enhancing the Scan-to-BIM process in various ways:
- Automated Object Recognition: AI algorithms can identify and classify objects within the point cloud data. This includes recognizing structural elements like beams and columns and architectural components such as windows and doors. This automated recognition speeds up the modeling process significantly.
- Data Cleanup: AI can assist in cleaning up the point cloud data by identifying and removing artifacts, such as shadows or reflections, that might distort the accuracy of the 3D model.
- Semantic Understanding: AI can provide semantic understanding to the model, allowing it to differentiate between similar objects. For example, it can distinguish between load-bearing walls and non-structural partitions, which is essential for structural analysis.
- Change Detection: AI algorithms can compare multiple scans of the same structure taken at different times to detect any changes or deformations. This is crucial for monitoring structural integrity in the long run.
- Efficient Annotation: AI can automatically annotate the 3D model with relevant information, such as material specifications, component names, and dimensions, reducing the need for manual data entry.
- Clash Detection: AI can detect clashes within the BIM model, identifying conflicts or collisions between building components, and reducing potential construction errors and rework.
The Role of ML in Scan-to-BIM
Machine Learning complements AI in the Scan-to-BIM process in the following ways:
- Pattern Recognition: ML algorithms can learn from a vast dataset of scanned structures to recognize patterns and trends in the data. This enables better object recognition and classification, even for complex and unique architectural features.
- Predictive Modeling: ML can be used to create predictive models for building performance, energy efficiency, and maintenance requirements. By analyzing historical data and real-time sensor inputs, ML can help optimize building operations.
- Data Fusion: ML algorithms can integrate data from various sources, such as 3D scans, 2D drawings, and sensor data, to create a comprehensive and accurate BIM model that reflects the real-world conditions of a building.
- Semi-Automation: ML can semi-automate the modeling process by suggesting and generating 3D elements based on scanned data, reducing the manual effort required.
- Optimization: ML can optimize the placement of building components for energy efficiency, structural stability, and cost-effectiveness, resulting in more sustainable and cost-efficient designs.
Benefits of AI and ML in Scan-to-BIM
The integration of AI and ML into the Scan-to-BIM process offers several significant benefits:
- Accuracy: AI and ML algorithms significantly improve the accuracy of the 3D models by reducing human error in object recognition, data cleanup, and annotation.
- Speed: Automation and semi-automation provided by AI and ML speed up the modeling process, allowing for quicker project turnaround times.
- Cost Reduction: By automating repetitive tasks and reducing the need for manual data entry, AI and ML help lower labor costs and minimize errors that could lead to costly construction issues.
- Better Decision-Making: The rich data generated by Scan-to-BIM, coupled with AI and ML analytics, provides valuable insights for architects, engineers, and project managers, enabling better decision-making at every project stage.
- Sustainability: ML-driven optimization can lead to more sustainable building designs, reducing energy consumption and environmental impact.
- Lifecycle Management: AI and ML enable the creation of BIM models that can be used throughout a building’s lifecycle, from design and construction to operation and maintenance.
Challenges and Considerations
While AI and ML offer substantial benefits to the Scan-to-BIM process, there are also challenges and considerations to keep in mind:
- Data Quality: The accuracy of AI and ML algorithms heavily depends on the quality of the input data. Low-quality scans or incomplete data can lead to inaccuracies in the BIM model.
- Training Data: Machine Learning models require extensive training data to perform effectively. This data needs to be diverse and representative of the structures being scanned.
- Privacy and Security: Handling sensitive building data requires robust security measures to safeguard against unauthorized access and prevent data breaches.
- Integration: Integrating AI and ML into existing workflows and software systems can be complex and may require adjustments to established processes.
Conclusion
Integrating Artificial Intelligence and Machine Learning into the Scan-to-BIM process has revolutionized how buildings and infrastructure are designed, constructed, and maintained. These technologies enhance accuracy, efficiency, and decision-making throughout the building lifecycle. While there are challenges to overcome, the benefits of AI and ML for Point Cloud to BIM Services are undeniable, making them indispensable tools for architects, engineers, and construction professionals in the modern era. As technology continues to evolve, we can expect even more significant advancements in the field of Scan-to-BIM, further reshaping the future of construction and architecture.